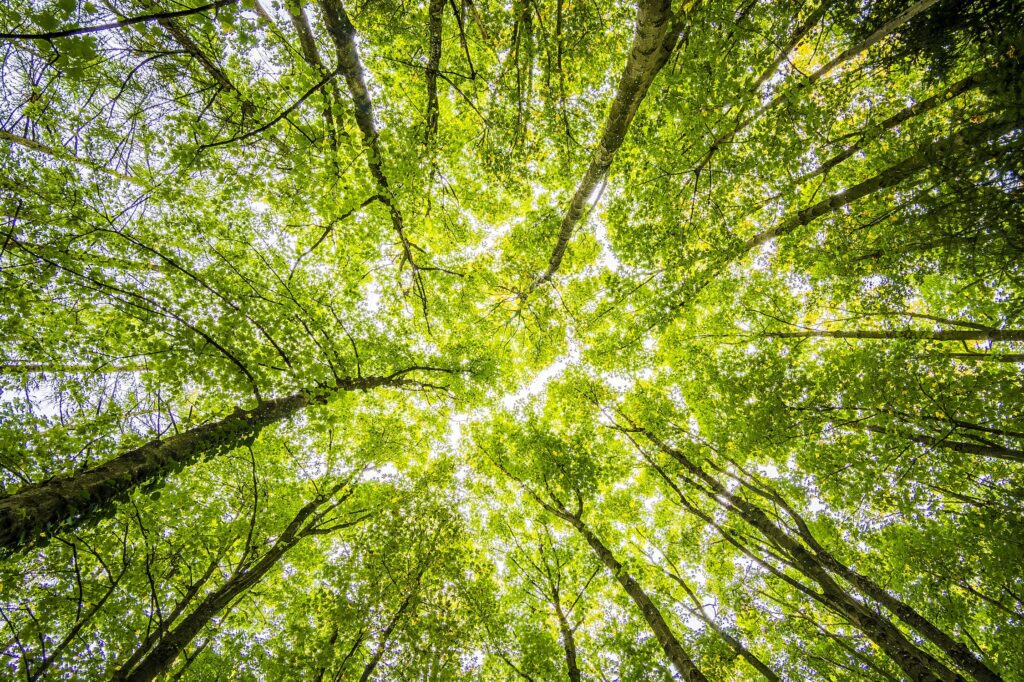
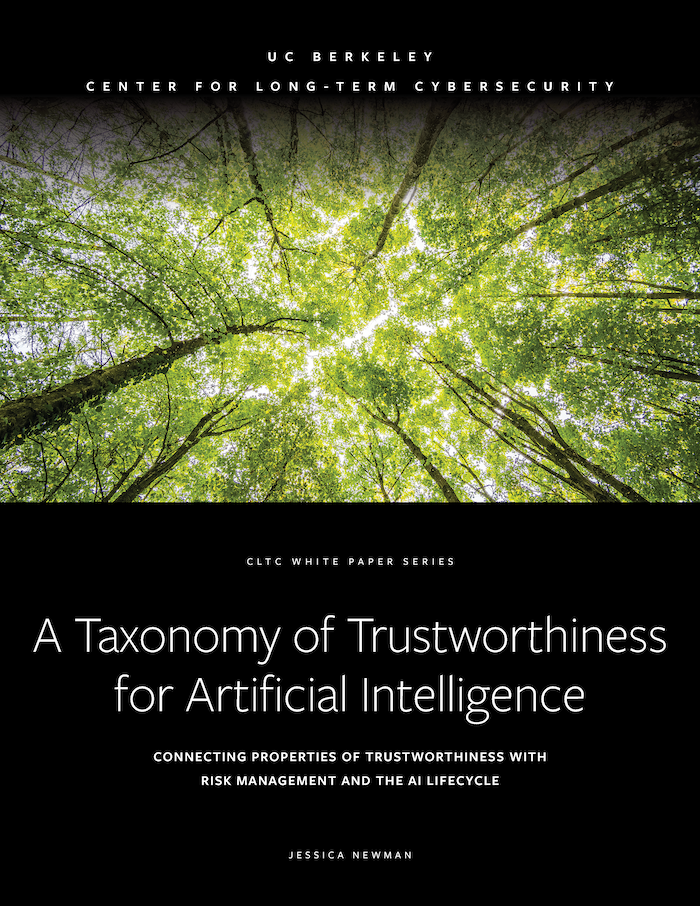
The National Institute of Standards and Technology (NIST) has developed an AI Risk Management Framework (RMF) intended to promote trustworthy artificial intelligence (AI). In this report, we introduce a taxonomy of trustworthiness for artificial intelligence that is intended to complement and support the use of the NIST AI RMF.
The taxonomy includes 150 properties of trustworthiness for AI. Each property builds upon a relevant “characteristic of trustworthiness” as defined by NIST in the AI RMF. NIST’s characteristics of trustworthiness include: valid and reliable; safe, secure, and resilient; accountable and transparent; explainable and interpretable; privacy-enhanced; and fair with harmful bias managed.
The taxonomy is organized by the seven stages of the AI lifecycle depicted in the NIST AI RMF:
- Plan and Design
- Collect and Process Data
- Build and Use Model
- Verify and Validate
- Deploy and Use
- Operate and Monitor
- Use or Impacted By
Our hope is that this framework supports usability by connecting the taxonomy more closely to actual product cycles and workflows. We also hope to provide ideas about possible ways to connect the NIST AI RMF Core to the AI lifecycle.
Within each stage of the lifecycle, the taxonomy includes NIST’s seven characteristics of trustworthiness. These categories are further broken down to include all the properties of trustworthiness that are relevant at that stage of the lifecycle.
We also include an eighth characteristic of trustworthiness in the taxonomy, which varies slightly from the NIST AI RMF. The additional characteristic is “Responsible Practice and Use.” The NIST AI RMF recognizes its importance and states that “AI risk management can drive responsible uses and practices,” but does not include it as a characteristic of trustworthiness. In this paper, we include it as a crosscutting characteristic of trustworthiness because we find that it serves a critical role in highlighting the interconnected nature of AI technologies with the people, organizations, and structures that are designing, building, and deploying them. We use Responsible Practice and Use in this taxonomy to promote consistent understanding of AI as a sociotechnical system, situated within structures of practice and use.
Each property is accompanied by a set of questions to guide initial thinking. For example, the “Data Protection” property includes the question, “How will we use encryption, differential privacy, federated learning, data minimization, and/or other best practices to protect data?” The questions are formulated in this future-oriented way (and not as “Have we . . . ?”) because they are intended to serve as a tool to spark further discussion and action, rather than as a checklist or a scorecard.
Each property included in the taxonomy is also tagged with a set of subcategories from the NIST AI RMF. These subcategories represent the most relevant sections of the NIST AI RMF Core framework. Reviewing these subcategories will point a reader to helpful resources and tools to address the property. A small number of the listed subcategories (in most cases just one or two) are bolded to emphasize that they are likely to be particularly helpful or a good place to start. There may be additional subcategories not listed here that are also relevant, depending on the context of an AI system’s development and use.
Finally, the taxonomy was developed to be useful for understanding a full spectrum of AI systems, including those that have limited engagement with people, which have typically been underemphasized in considerations of AI trustworthiness. A subset of the properties of trustworthiness in the taxonomy are likely to only be relevant to AI systems that are human-facing, which may engage directly with human users or operators, make use of human data, or inform human decision-making. These properties are marked in the table with an asterisk after their name. Properties that do not have an asterisk are likely to be relevant to AI systems across the spectrum of human-AI engagement.
This taxonomy aims to provide a resource that is useful for AI organizations and teams developing AI technologies, systems, and applications. While it is designed specifically to assist users of the NIST AI RMF, it could also be helpful for people using any kind of AI risk or impact assessment, or for people developing model cards, system cards, or other types of AI documentation. It may also be useful for standards-setting bodies, policymakers, independent auditors, and civil society organizations working to evaluate and promote trustworthy AI.
This resource includes the Taxonomy of Trustworthiness for Artificial Intelligence, which is discussed in more detail in the full paper available here: https://cltc.berkeley.edu/publication/a-taxonomy-of-trustworthiness-for-artificial-intelligence/. The full paper also includes discussion of the term “trustworthy AI,” compares multiple existing frameworks for trustworthy AI that informed this work, introduces the concept of properties of trustworthiness for AI, and includes in the appendix connections to international AI standards and a list of the properties of trustworthiness without segmentation by lifecycle stage.