Are you an instructor who teaches an introductory machine learning (ML) course Developed by the University of California, Berkeley’s Center for Long-Term Cybersecurity (CLTC), this Fairness Mini-Bootcamp is a week-long curriculum designed to teach students how to detect, identify, discuss and address bias in real-world machine learning algorithms. This page provides all the materials you’ll need to teach these topics to students in your classes.
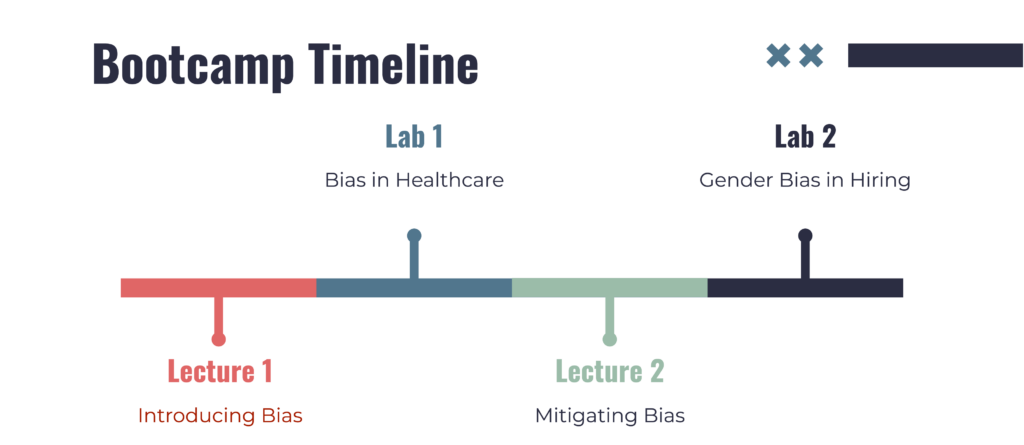
About
The bootcamp includes two lectures introducing machine learning bias and fairness, along with labs that delve into how these algorithms are situated in larger social contexts, prompting students to discuss who designs these algorithms, who uses them, and who gets to decide what it means for algorithms to be working properly.
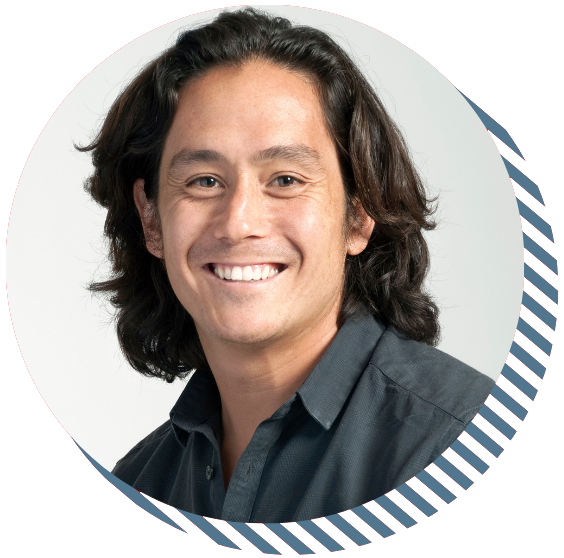
“The Daylight Lab ML Fairness Bootcamp was excellent. It gave
Joshua Blumenstock
my students a structured overview of common sources of bias,
and frameworks for reasoning about fairness in applied machine
learning settings. Students especially appreciated the hands-on labs,
which provided an opportunity for hands-on experience with the ways
in which standard ML algorithms can fail in real-world environments.”
Associate Professor, UC Berkeley School of Information
This course plan is designed to help students answer questions like: If you have an algorithm in front of you, how do you know if that algorithm is biased? How is it biased? And what do those biases mean in practice?
These course materials are licensed under the Creative Commons CC BY-NC-SA 4.0 license, so you can copy and modify them for non-commercial use; simply attribute the original source.
Let us know how you end up using these materials,
and we welcome your questions, ideas, and other feedback.
We are eager to improve this offering.
Lecture 1: Identifying Bias
This lecture introduces algorithmic bias and gives real-life examples of how algorithmic bias has harmed people.
Topics addressed
- What is the problem?
- Why is it a big deal?
- How does it happen?
- What can we do?
Key ideas
- Machine learning algorithms can exhibit bias against people whose characteristics have served as the basis for systematically unjust treatment in the past. This bias can emerge for a variety of reasons, and can be so severe as to be illegal.
- Bias in machine learning algorithms is both a social and a technical problem. There are no technical “fixes,” though technical tools can help us identify bias and reduce its harmfulness.
- Do not remove sensitive features (like race and gender) from your data. That makes it impossible to understand how your algorithm treats people with those characteristics, or if it exhibits a bias against them.
Topics for breakout discussions & activities:
- What are some examples of machine learning bias you’ve heard about?
- What are some uses for machine learning where bias is a particular concern?
- In the healthcare example, who got to decide when the algorithm was fair enough?
- Why is it dangerous to remove race (or other sensitive attributes) from your training set?
Recommended assigned reading before the lecture:
Obermeyer, Powers, Vogeli, and Mullainathan. 2019. Dissecting racial bias in an algorithm used to manage the health of populations. Science
Lab 1: Identifying racial bias in a health care algorithm
To effectively manage patients, health systems often need to estimate particular patients’ health risks. Using quantitative measures, or “risk scores,” healthcare providers can prioritize patients and allocate resources to patients who need them most. In this lab, students examine an algorithm widely used in hospitals to establish quantitative risk scores for patients. (At the time we developed this lab, this algorithm was applied to 200 million patients in the U.S. every year!). Students will discover how this algorithm embeds a bias against Black patients, undervaluing their medical risk relative to White patients.
Resources
- Watch a video walkthrough of this lab with a student.
- See a primer that covers the Python knowledge required to complete the programming version of this lab.
Lecture 2: Ameliorating Bias
Topics addressed
- How do experts talk about bias?
- What metrics can describe bias?
- How do we make biased algorithms less harmful?
- Issues in AI safety beyond fairness and bias
Key ideas
- Metrics like disparate impact can help describe the extent of bias toward particular groups.
- Methods like fairness constraints can ameliorate the impact of bias, even with a fixed dataset.
- Fairness is a sociotechnical problem; no technical “fix” can “solve” bias in machine learning
Recommended reading
Reading: Mulligan, Kroll, Kohli & Wong. 2019. This Thing Called Fairness: Disciplinary Confusion Realizing a Value in Technology. Proceedings of the ACM on Human-Computer Interaction.
(Optional) Lab 2: Ameliorating gender bias in a hiring algorithm
Various companies (including Amazon) have attempted to use machine learning to automate hiring decisions. However, when these algorithms are trained on past hiring decisions, they are likely to learn human biases: in this case, encoding a pay gap between men and women. From a social and ethical standpoint, we want to remove or minimize this bias so that our models are not perpetuating harmful stereotypes or injustices. In this lab, we take a dataset in which prior hiring decisions have adversely impacted women, and show how applying fairness constraints can ameliorate the effect of this impact, making it less harmful. We also prompt students to consider when, whether, and to what extent machine learning ought to be applied to hiring decisions.