The Center for Long-Term Cybersecurity has selected two UC Berkeley graduate students to receive the 2023 Cal Cybersecurity Research Fellowship. This year’s fellows are Marsalis Gibson, a PhD student in the UC Berkeley Department of Electrical Engineering and Computer Science (EECS), and Sarah Barrington, a PhD candidate in the UC Berkeley School of Information.
“We are excited to support these two PhD students as they pursue important research on the frontiers of artificial intelligence-enabled cybersecurity,” said Ann Cleaveland, Executive Director of CLTC.
Awarded annually since 2019, the Cal Cybersecurity Research Fellowship was launched through the generous support of a Cal alumnus. The Fellowship award provides up to $30,000 to UC Berkeley students or postdoctoral scholars to pursue cybersecurity research in security-related fields.
Gibson and Barrington were selected from a pool of applicants from a range of UC Berkeley departments. As detailed in the call for applications, this year’s fellowship is specifically focused on “supporting scholars exploring how artificial intelligence technologies can help automate and amplify cyberspace capabilities, for example through automated vulnerability detection, attack discovery, or other AI-enabled cybersecurity practices. We are hoping to facilitate projects that can meaningfully push the envelope on cybersecurity capabilities through the integration of AI and automation.”
Gibson’s research broadly focuses on the security, safety, and integrity of AI control systems. He is investigating “machine-learning-enabled defense techniques on control-based navigation systems, like autonomous driving, that will defend against attacks which have potential downstream effects on controls.” Gibson is advised by Professors Claire Tomlin and Shankar Sastry; he has affiliations with Berkeley’s Artificial Intelligence Research (BAIR) Lab and the Institute of Transportation Studies (ITS).
Barrington’s research spans digital forensics, computer vision, and artificial intelligence. For her fellowship project, entitled “The Deep-fake Captcha: A Multimodal Approach to Real-Time Deep-Fake Detection,” she will “develop a multimodal AI pipeline that can serve as a deep-fake ’Captcha,’ trained upon seconds of audio-visual content for an individual, rather than hours of historic footage, and agnostic of what generative models may be used.” Barrington works with Hany Farid, Professor in the UC Berkeley Department of Electrical Engineering & Computer Sciences and the School of Information.
Barrington and Gibson join past honorees of the Cal Cybersecurity Fellowship who have conducted research on such cybersecurity topics as improving user adoption of multi-factor authentication, making “internet of things” technologies more private, and detecting attacks on cyber-physical systems interacting with 5G. (More information about previously awarded Cal Cybersecurity Research Fellowships can be found here.) Abstracts from Gibson’s and Barrington’s research proposals are included below (edited for length).
Sarah Barrington
The weaponization of deep-fake technologies has emerged as a prevalent threat to modern online safety, challenging society’s ability to verify trusted information. The increasing sophistication of deep-fake technologies has led to the emergence of problems ranging from the creation of fake videos of CEOs and world leaders to the dissemination of revenge porn. Now, we face the so-called ‘liar’s dividend’ of misinformation, in which the truth is discredited by the uncertainty around what is real and what is not.
Building upon the limitations of prior work, our proposed research will use combined features in order to develop a multimodal AI pipeline that can serve as a deep- fake ’Captcha’, trained upon seconds of audio-visual content for an individual, rather than hours of historic footage, and agnostic of what generative models may be used. The expected outcomes of this work will be highly impactful in the verification and authenticity of streaming content, empowering internet users worldwide to rebuild trust in what they see on their screens.
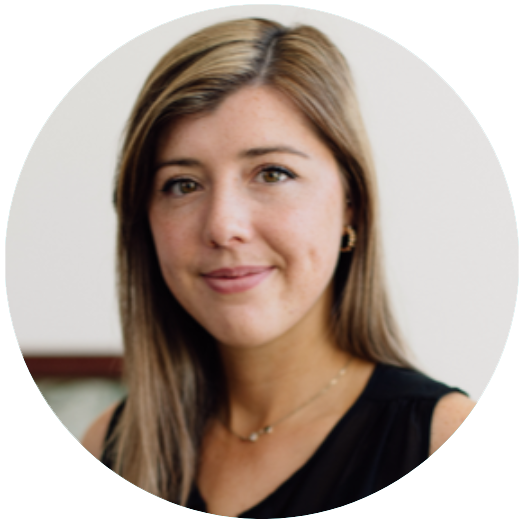
Marsalis Gibson
Many new technologies, such as autonomous driving, are actively being developed and deployed. In the past decade/years, cybernetic attacks to technological services used by humans have significantly increased. These attacks, especially in the context of autonomous driving, pose a threat to human safety…. While detecting and preventing these attacks can be challenging, it may be possible to leverage Machine Learning (ML) to develop principled defenses for common vulnerabilities and improve vehicles’ overall resilience. We propose to study ML-enabled defense techniques on control-based navigation systems, like autonomous driving, that will defend against attacks which have potential downstream effects on controls.
First, we will conduct an extensive “threat analysis and risk assessment” for autonomous vehicles that considers attacks on ML components, attacks on sensors, vehicle-to-everything network attacks, and potential malware. This assessment will evaluate how effective threats are at decreasing a vehicle’s overall driving ability and how effective they are at leading a vehicle to violating some driving safety specification. Next, we will propose 2-3 ML-based defense techniques — ML based intrusion detection and ML based malware analysis — and analyze how effective these defenses are at mitigating the impact of the threats being studied. This project will be among the first to conduct a threat analysis and risk assessment of possible attacks on automated vehicles with ML, identify broad classes of vulnerabilities, and produce broad ML-based security defenses using intrusion detection and analysis. We expect our work to be the pioneer in preventing cyber attacks on cyber-physical systems, which are rapidly spreading in our society due to the increase of user-oriented autonomous technologies with integrated ML.
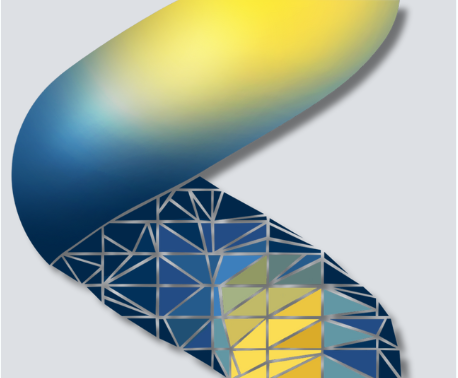