The continual improvement of models for author attribution—the task of inferring the author of an anonymized document—indicates potential benefits but also substantial risks in the context of privacy and cybersecurity. Such improvements pose particular threats to whistleblowers and other individuals who might have strong political or security-related reasons for wanting to conceal their identities. Even when the candidate set is too large to identify a specific author, it is hypothetically possible to determine sensitive attributes about an individual that could be used for detrimental or biased purposes. The primary goals of this project are to provide a thorough review of prior research into author obfuscation and to suggest new approaches for improving current systems. In particular, we will create a benchmark dataset to better measure the performance of current and future models for adversarial stylometry while also proposing new neural methods specifically tailored to this task.
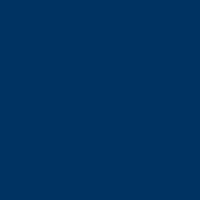
Grant /
January 2020
Obscuring Authorship: Neural Methods for Adversarial Stylometry and Text-Based Differential Privacy
By