Machine learning provides valuable methodologies for detecting and protecting against security attacks at scale. However, a machine-learning algorithm used for security is different from other domains because in a security setting, an adversary will try to adapt his behavior to avoid detection. This research team will explore methodologies for improving the robustness of a machine-learning classifier. This work will improve the understanding of the brittleness of machine-learning solutions and provide guidelines for improvement.
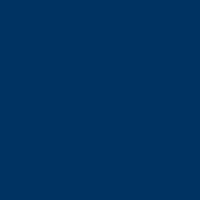
Grant /
January 2020