Since 2019 CLTC has provided a Cal Cybersecurity Research Fellowship, made possible through the generous support of Tim M. Mather, CLTC External Advisor and a Cal alumnus (Class of ´81).
The outsized results of these awards on both student recipients and research outcomes inspired CLTC to formalize a Cal Cybersecurity Research Fellowship Fund, inviting the broader community to directly support talented students like those profiled below:
- 2025 honorees Changcheng Fan and Zhun Wang
- 2024 honoree Caseysimone Ballestas and DARPA AI Cyber Challenge Research Team
- 2023-24 honoree Gowri Swamy;
- 2023 honorees Sarah Barrington and Marsalis Gibson;
- 2022-23 honorees Team Kohana;
- 2022 honorees Emma Lurie and Conor Gilsenan;
- 2021 honorees Tanu Kaushik and Ji Su Yoo;
- 2020 honoree Nathan Malkin; and
- 2019 inaugural honoree, Matt Olfat.
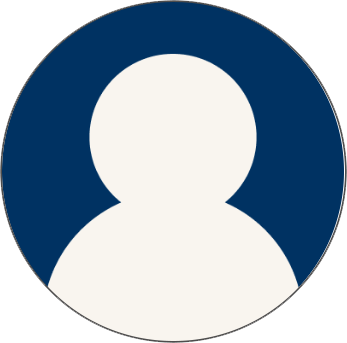
Changcheng Fan
2025 Fellow, Postdoctoral Researcher, UC Berkeley
Internet censorship is a critical human rights issue, as the internet has rapidly become the primary mode of communication globally. While many censorship circumvention tools exist, they are frequently targeted by censors, leaving users vulnerable to sudden shifts in censorship strategies and resulting in drastic loss of access. In response to this challenge, I propose Avenger, a censorship speculation platform powered by artificial intelligence. Avenger uses AI algorithms to generate plausible censorship strategies that researchers can use to enhance and refine obfuscation protocols preemptively, rather than reactively. By producing efficient, minimal programs that could feasibly be implemented by censors, Avenger allows circumvention developers to anticipate and counteract future censorship mechanisms. The platform has already demonstrated its effectiveness in preliminary studies, where we successfully identified a previously unknown blocking attack against the Trojan circumvention proxy with an accuracy of 99.764% and a false positive rate of 0.0. Avenger holds the potential to revolutionize how the circumvention community prepares for and responds to censorship threats, ultimately strengthening internet freedom for users worldwide.
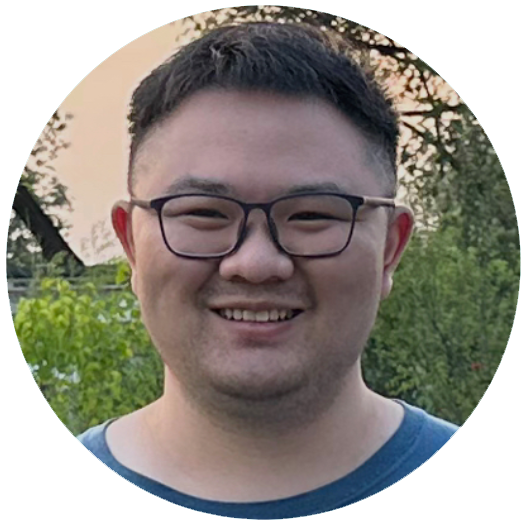
Zhun Wang
2025 Fellow, PhD Student, Electrical Engineering and Computer Science, UC Berkeley
Large language models (LLMs) such as ChatGPT have greatly advanced coding tasks but often fail to generate secure code. Current approaches to improving code security, relying on fine-tuning, struggle with robustness and generalizability. This proposal explores LLM interpretability to enhance secure code generation. By employing bottom-up (e.g., sparse autoencoders) and top-down (e.g., representation engineering) techniques, we aim to understand how LLMs internally represent code properties and security across tasks and vulnerability types. We will study training dynamics using model checkpoints and smaller LLMs to assess how these representations develop during pre-training and fine-tuning. Building on these insights, we propose advanced monitoring and control mechanisms to detect, intervene, and guide code generation in real time. Techniques such as representation engineering and representation intervention will enable precise manipulation of the generation process. We also plan to refine fine-tuning methods, emphasizing internal feature control to improve security comprehensively. This work seeks to create a robust framework for secure and reliable code generation in LLMs.

Caseysimone Ballestas
2024 Fellow, PhD Student, Mechanical Engineering, UC Berkeley
This research addresses a significant gap in cybersecurity knowledge among manufacturing design engineering professionals tasked with designing Industry 4.0’s manufacturing environments. It aims to understand how design decisions in the early design phases can minimize the attack surface area of dynamic manufacturing environments (DMEs) — factories with cyber-physical and IoT technologies. By extending our collaboration with Oak Ridge National Laboratory (ORNL), and with the support of this funding, we aim to provide insights critical for enhancing cybersecurity in Industry 4.0. Our central research question guiding this work is: How do early-stage design decisions influence the emergence of vulnerabilities and expansion of attack surfaces within the context of remote monitoring and control of subtractive machining at ORNL.
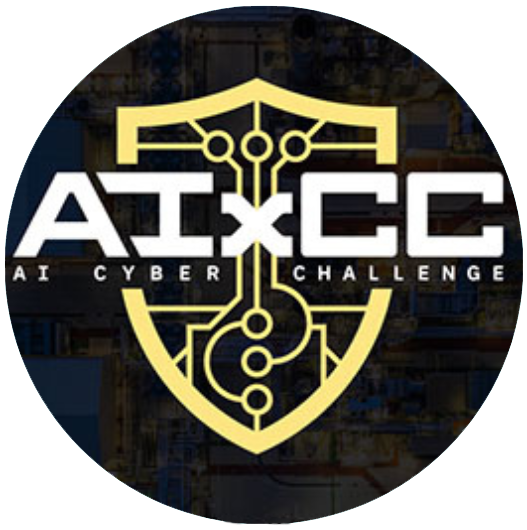
DARPA AI Cyber Challenge Research Team
A CodeLM Automated Repair Program with Analysis, Planning, and Control
Current implementations of vulnerability detection and automated code repairs have been beneficial to corporations and governments that develop applications that may be susceptible to vulnerabilities. Even though learning-based solutions have exceeded the current State-of-the-Art automated repair methods, these systems still suffer from low fault detection accuracy the “overfitting problem,” and computational inefficiency. Therefore, to address these problems, we propose to build an automated repair program that generates repairs in 2 steps, where the first step, Vulnerability Detection, identifies the vulnerability’s location; and the second step, Patch Generation, finds a patch that adequately fixes the vulnerability while maintaining its original functionality, style, and readability. Specifically, vulnerabilities will be detected using a model that processes static and dynamic information for context, while patches are produced by utilizing one Code LM to produce patching plans in the form of instructions then using another Code LM to follow the instructions and execute code changes. To optimize the system for competition, we will evaluate our system using metrics on both vulnerability detection and patching that reflect accuracy, effectiveness, acceptability, and code size. We finally evaluate any risks that may pose within our design, along with identifying mitigation strategies that may resolve these issues.
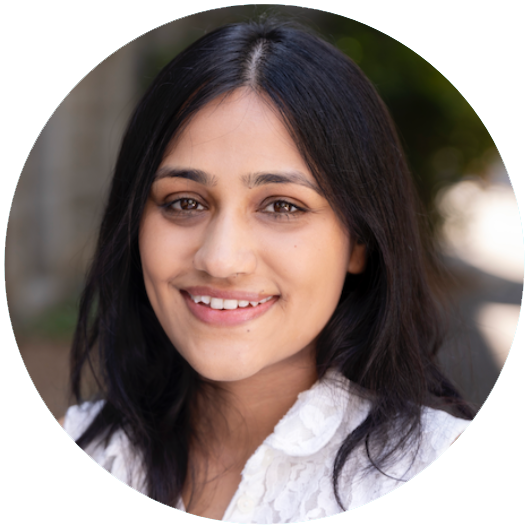
Gowri Swamy
2023-24 Fellow, UC Berkeley School of Information
With the advent of AI-generated media, it is now easier, faster, and cheaper to spread misinformation to a larger audience. However, policies and regulations for online governance are oftentime restricted due to the chance of not abiding by the constitutional right to freedom of speech. This empirical study aims to investigate the ongoing and turbulent partnership between social media platforms and content moderation, specifically taking a look at human perceptions of free speech and how they may (or may not) change when considering whether the perpetrator of misinformation is or is not human.
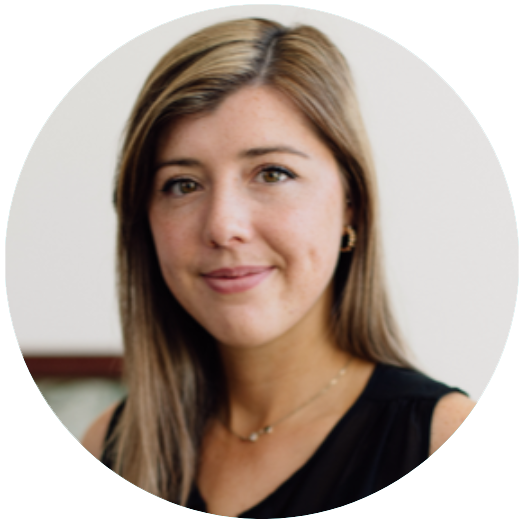
Sarah Barrington
2023 Fellow, UC Berkeley School of Information
The weaponization of deep-fake technologies has emerged as a prevalent threat to modern online safety, challenging society’s ability to verify trusted information. Building upon the limitations of prior work, our proposed research will use combined features in order to develop a multimodal AI pipeline that can serve as a deepfake ’Captcha’, trained upon seconds of audio-visual content for an individual, rather than hours of historic footage, and agnostic of what generative models may be used.
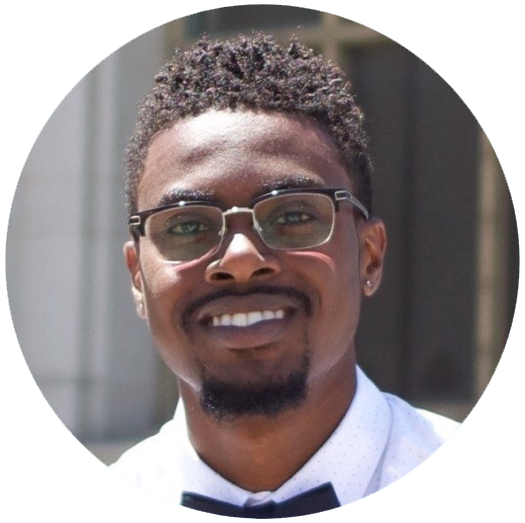
Marsalis Gibson
2023 Fellow, UC Berkeley Department of Electrical Engineering and Computer Science (EECS)
Studying ML-based intrusion detection and analysis against common attacks in autonomous driving.
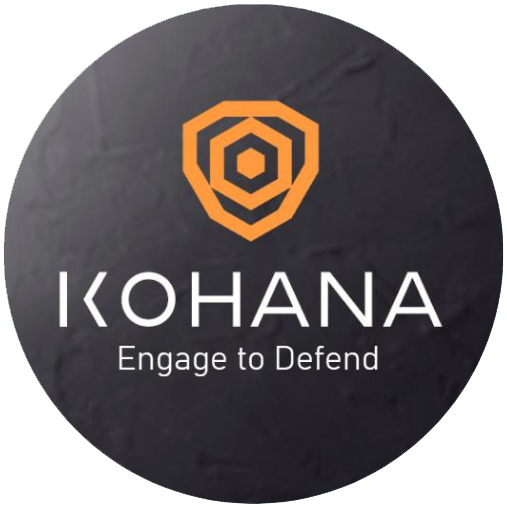
Team Kohana
2022-23 Fellows, UC Berkeley School of Information
Kohana is a distributed deception technology focused on protecting cloud assets through adversary engagement. It is designed to help customers operationalize their MITRE Engage™ playbooks, operating with the adversary engagement premise that the adversary only needs to be wrong once for us to detect and deny cyber threats.
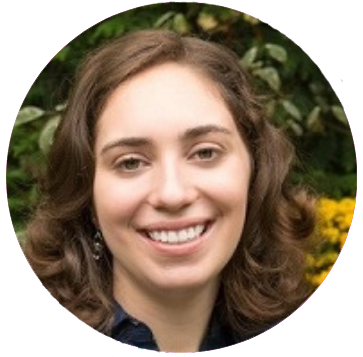
Emma Lurie
2022 Fellow, UC Berkeley School of Information
How policy choices of platforms and government agencies shape the online election information infrastructure, and how related misinformation is linked to voter disenfranchisement — particularly among marginalized communities that already have historical distrust in the election process.
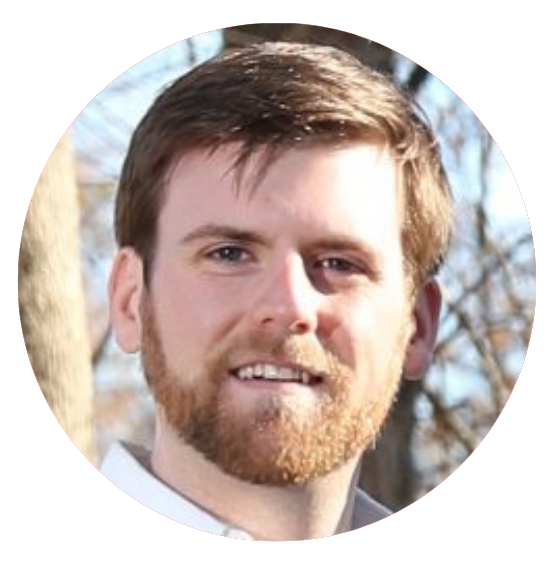
Conor Gilsenan
2022 Fellow, UC Berkeley Department of Electrical Engineering and Computer Sciences
Improving usability and account recovery mechanisms in adoption and acceptance of multi-factor authentication.
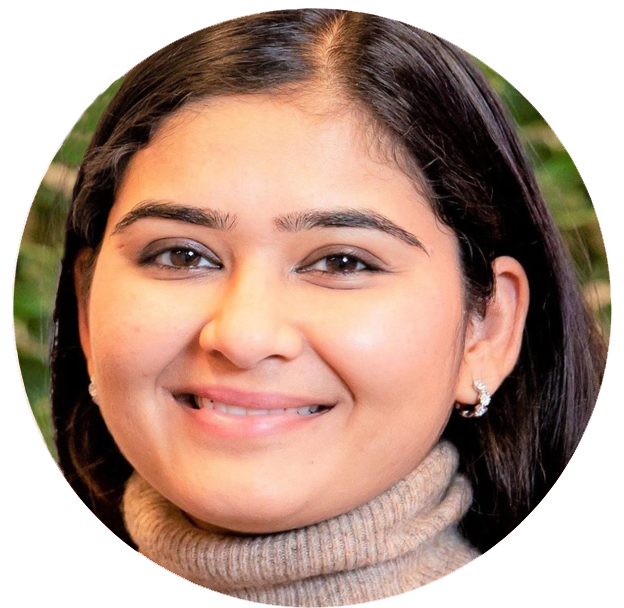
Tanu Kaushik
2021 Fellow, UC Berkeley School of Information
Understanding threats posed by adversarial machine learning and the detection, protection, response, and recovery mechanisms for known attack techniques.
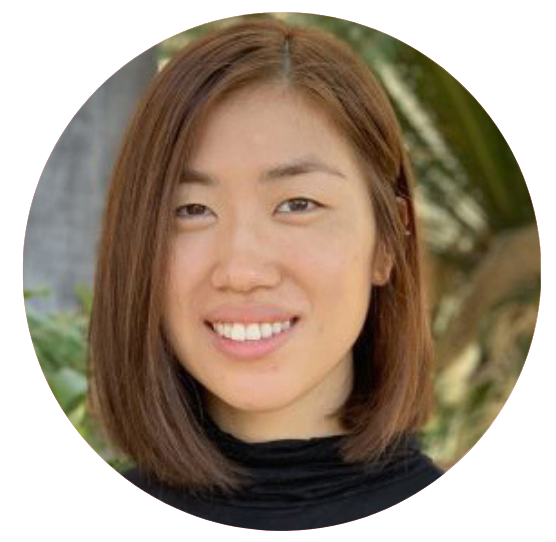
Ji Su Yoo
2021 Fellow, UC Berkeley School of Information
How the message and identity of the messenger impacts reception of misinformation corrections.
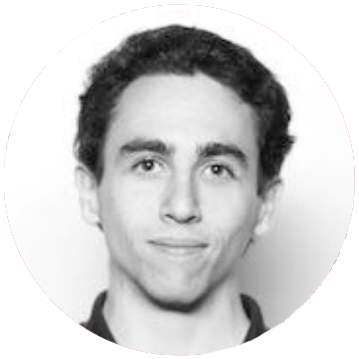
Nathan Malkin
2020 Fellow, UC Berkeley Department of Electrical Engineering and Computer Sciences
Making the Internet of Things technology more private — and privacy controls more equitable — for marginalized groups.
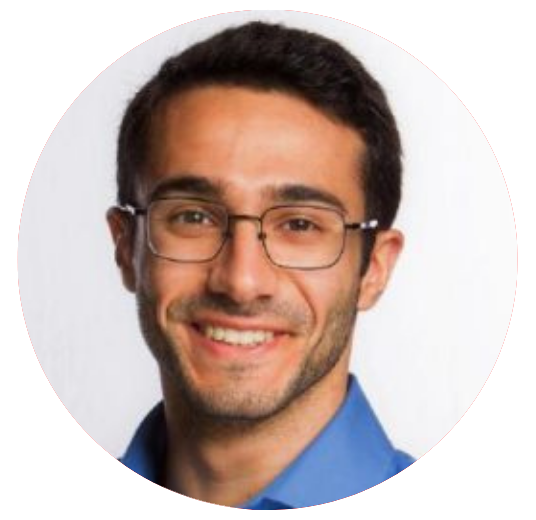
Matt Olfat
2019 Fellow, UC Berkeley Department of Industrial Engineering and Operations Research
Detecting attacks on cyber-physical systems interacting with 5G.