
Developers of artificial intelligence (AI) often struggle with making their models “explainable,” i.e., ensuring that users of the system understand how or why an output or decision was made. A new white paper from the Center for Long-Term Cybersecurity explores diverse approach to explainable artificial intelligence (XAI), focusing on one approach in particular: counterfactual explanations, or CTEs.
The paper, “Improving the Explainability of Artificial Intelligence: The Promises and Limitations of Counterfactual Explanations,” was authored by Alexander Asemota, a PhD candidate in statistics at UC Berkeley. Asemota conducted his research on counterfactual explanations during his term as a fellow in the AI Policy Hub, an interdisciplinary initiative that trains UC Berkeley researchers to develop effective AI governance and policy frameworks.
“As AI rises in prominence across domains, it is crucial that companies, governments, and the public understand how AI is impacting decision-making,” Asemota writes. “However, there continues to be a dearth of guidance on how decisions made by algorithmic systems should be explained to those affected by them. This paper seeks to offer some perspective on counterfactual explanations, a methodology that has the potential to greatly improve access to recourse for algorithmic subjects.”
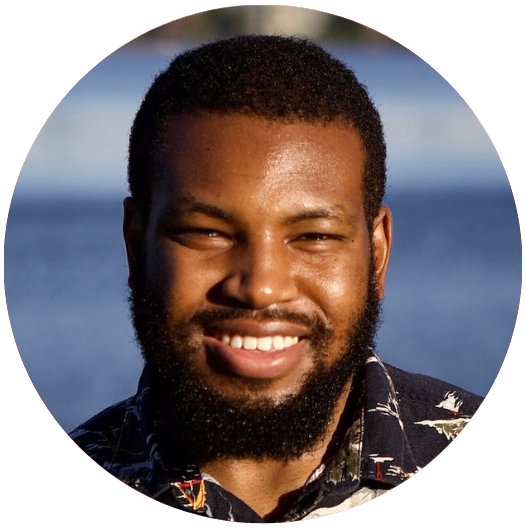
Counterfactual explanations, Asemota explains, are “intuitive for users because they describe how changing the factors that went into an algorithm-based decision would lead to a different output.” He offers the example of an applicant denied admission to a university: a counterfactual explanation might recommend that the applicant increase their test scores or take additional courses to improve their chances.
Still, while CFEs offer some improvements over other explanation methods, they still have significant limitations, Asemota writes. Companies, lawmakers, researchers, and regulators must keep these limitations in mind when considering how and when to use CFEs. Asemota provides specific recommendations for different stakeholders in shaping AI and AI policy, including regulators, lawmakers, companies and researchers.
“Counterfactual explanations are promising as they provide specific recommendations to the user in a format that does not require significant knowledge of AI.”
Asemota suggests that regulators of AI technologies should, at least for now, refrain from requiring AI developers to provide counterfactual explanations, due to deficiencies in existing methodologies. They should, however, support the development of frameworks for explainability, and collaborate with open-source developers to create robust libraries for models, tools, and methodologies that support explainability.
Lawmakers, meanwhile, should require reporting on explainability for high-stakes domains such as finance and medicine. They should also require that technology makers disclose the use of AI systems, ensuring that algorithmic subjects are aware of when and how AI/ML is being used for decisions that affect them.
Companies should continue to test the use of counterfactual explanations, including by comparing the recommendations provided by CFEs to observed data to evaluate their accuracy and effectiveness. They should also test and validate any methods for explainability that they intend to implement, and build rigorous and automatic evaluation structures into AI pipelines.
Asemota recommends that AI researchers collaborate with applied AI/ML practitioners to progress counterfactual explanations research. They should place higher emphasis on safety and explainability in AI research, and communicate the risks in using explainability methods developed through their research.
“The rise of AI/ML has led to a growing need for explainability and transparency from what are often opaque systems,” Asemota writes in the conclusion. “Counterfactual explanations are a promising tool in the pursuit of explainable AI, but CFEs have significant limitations. Regulators, legislators, private companies, and researchers all have a role to play in improving counterfactuals and increasing explainability in AI/ML more generally.”